Closed-Loop Data-Driven Decision Support Systems: What They Are and Why Your Organisation Needs Them
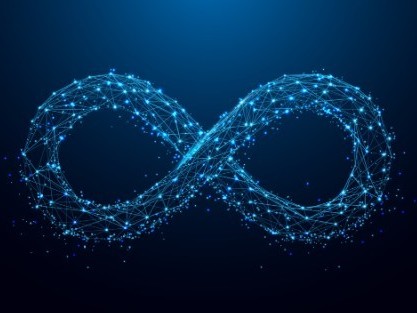
posted 10th February 2025
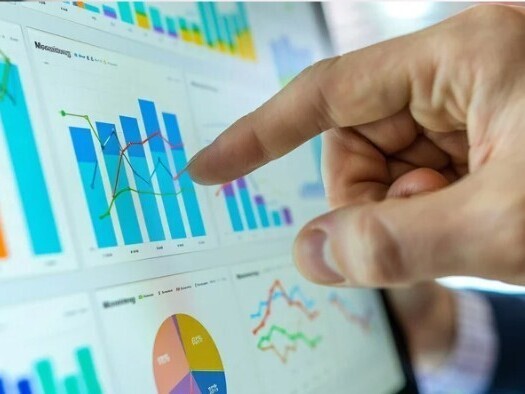
Closed-Loop Data-Driven Decision Support Systems: What They Are and Why Your Organisation Needs Them
Needless to say, organisations are increasingly relying on information to drive decisions. However, making decisions based on static or disconnected data can lead to suboptimal outcomes. This is where Closed-Loop (Data-Driven) Decision Support Systems (CLDSS) come into play. These systems allow organisations to continuously refine their decision-making processes, ensuring that every decision feeds back into the system to inform future actions.
What Are Closed-Loop Data-Driven Decision Support Systems?
At their core, CLDSS are operating model and underlying process and technical set ups that not only help organisations make decisions based on data but also continuously incorporate the outcomes of those decisions back into the system. This feedback loop enables a cycle of continuous learning, improvement, and refinement. Rather than simply informing decisions with data, CLDSS create a dynamic process of ongoing analysis and adjustment.
Real-World Examples of Closed-Loop Systems
-- Manufacturing Industry (General Electric): General Electric (GE) has implemented a closed-loop system in its industrial operations using its Predix platform. This system collects real-time data from manufacturing equipment, uses predictive analytics to guide maintenance schedules, and adjusts operations based on outcomes. If maintenance decisions lead to better performance, the system learns and adjusts its algorithms to make even better decisions in the future.
--Retail (Amazon): Amazon's recommendation engine is a prime example of a closed-loop decision support system. By analyzing purchase data and feedback from customers, Amazon adjusts its algorithms in real-time, offering better product recommendations to customers based on previous interactions. The system also tracks outcomes, measuring how users interact with recommended products, which refines the decision-making process to improve future suggestions.
--Healthcare (IBM Watson for Oncology): IBM's Watson for Oncology provides doctors with data-driven insights by analyzing a vast array of medical records, research, and patient data. Once a treatment plan is recommended, the system continues to track patient outcomes. These results are fed back into the system, allowing Watson to refine its future recommendations based on the effectiveness of previous decisions. This creates a feedback loop that enhances the system’s ability to suggest personalized treatment options.
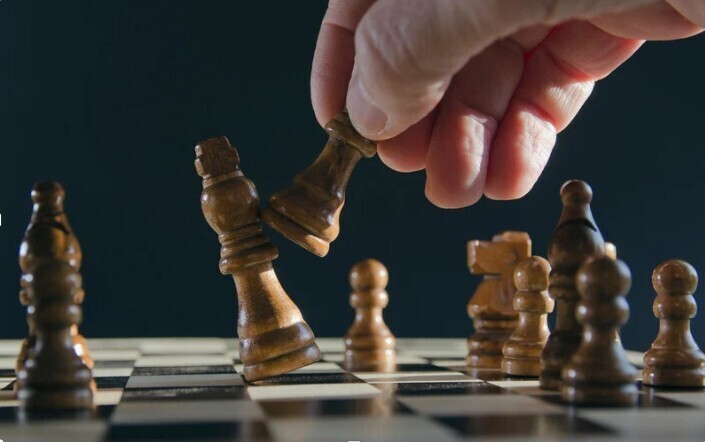
Why Organisations Should Strive Towards Closed-Loop Systems
CLDSS enable organisations to refine their processes in real-time, rather than waiting for periodic reviews and consensus by committee situations. The feedback loop of continuous improvement ensures that decisions are always based on the latest insights, leading to better outcomes.
The continuous flow of data also ensures that organisations can respond quickly to changes in the market, customer behaviour, or internal performance making them more agile and responsive. It allows for swift adaptation to evolving conditions, which is critical in today’s fast-paced business environments.
With CLDSS, decisions are based on the most accurate, up-to-date data available supporting informed decision-making. This removes reliance on gut feeling, unconscious cognitive biases or outdated information and ensures that decisions are more objective, data-driven, and effective.
What Are the Prerequisites for Operating Closed-Loop Systems?
Before organisations get too excited about CLDSS, a thorough and honest organisational audit is required. Not just technical, but that relating to policy, process and culture and the governance mechanisms controlling these elements. Overarching organisational strategies also need to be probed for their pragmatism and feasibility which can often cause discomfort in C-suites.
To implement and operate a CLDSS effectively, organisations need:
Integrated Data Infrastructure: The data landscape must be unified across systems, departments, and platforms. A robust data infrastructure allows seamless integration and real-time processing of data from different sources. This is said easily as a statement in an article but requires real committed invested to make a reality.
Advanced Analytics Capabilities: Beyond basic data reporting, organisations need predictive and prescriptive analytics to guide decision-making. These tools help in understanding not just what is happening, but also why it's happening and what will happen next. And to be predictive and prescriptive, organisations need to know where they're heading towards.
Feedback Mechanisms: Closed-loop systems rely heavily on continuous feedback. Clear processes for capturing, analysing, and acting on the outcomes of decisions are essential for refining and improving future strategies. This often can result in the feeling of 'loss of control' but feedback mechanisms can be iterative and then evolved to be more automated once confidence builds.
Cross-Functional Collaboration: Collaboration across departments is crucial to ensure that data is being shared and insights are considered from all relevant perspectives. Decision-making should be holistic, informed by a broad range of internal and external data.
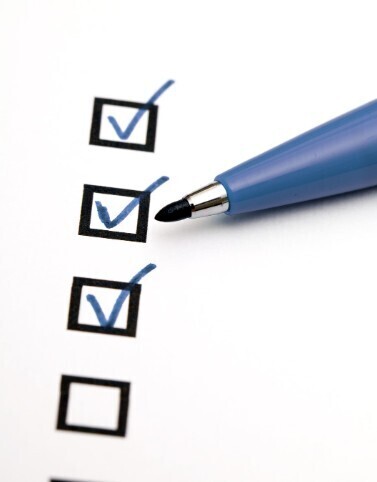
3 Key Steps to Moving Towards CLDSS
- Evaluate and Modernise Data Infrastructure: Assess the current state of your data infrastructure. Invest in technologies that allow for data integration, accessibility, and real-time processing. This may involve upgrading legacy systems or adopting cloud-based platforms. Technical debt is no small thing and can defeat the most enthusiastic of reformers. Play the long game, stay the course and modernise and future proof which means horizon scanning at the same time.
- Leverage Advanced Analytics: Implement tools that go beyond basic data analysis, incorporating predictive analytics and machine learning to guide decision-making. These tools can help forecast future trends and prescribe optimal courses of action. If in house expertise and knowledge does not exist in the areas of machine learning and advanced data engineering, spend the budget and buy in the talent.
- Implement Real-Time Feedback Loops: Design processes that capture the outcomes of decisions in real-time. This feedback should be integrated into the system to help refine future decisions. Automation and monitoring systems should be set up to immediately analyze the results of actions and adjust the decision-support system’s recommendations.
- Foster a Data-Driven Culture: For a CLDSS to succeed, it’s essential that all employees, from top management to operational teams, embrace a data-driven mindset. Create training programs, offer incentives for data-driven decisions, and break down silos to ensure that data is shared and used effectively across departments. This collaborative approach will enhance the system's effectiveness. This is also no small task. No more anecdotes. Every person in every meeting relating to strategic or operational matters should be used to asking 'What does our data tell us about this?'
How Dataqubed Can Help
At Dataqubed Ltd, we help organisations transition to data-driven decision-making by:
- Reforming System Infrastructure: We integrate legacy systems with modern platforms, enabling seamless data flow.
- Optimising Data Landscapes: We build real-time data warehouses and analytics pipelines to provide actionable insights.
- Transforming Operating Models: We work with your teams to create collaborative, data-centric decision-making frameworks that drive continuous improvement.
By partnering with Dataqubed, your organisation will not only embrace data as a strategic asset but also create a sustainable, closed-loop decision support system that fuels ongoing success.
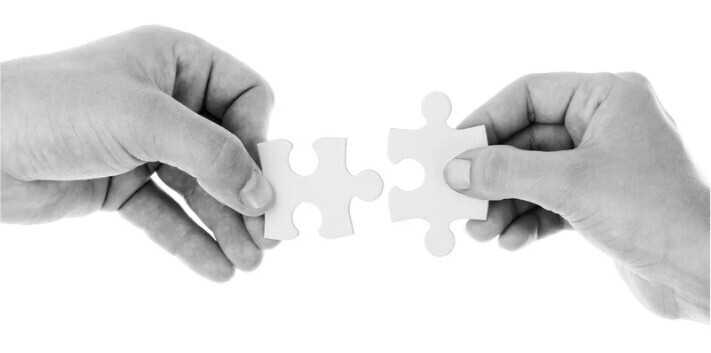